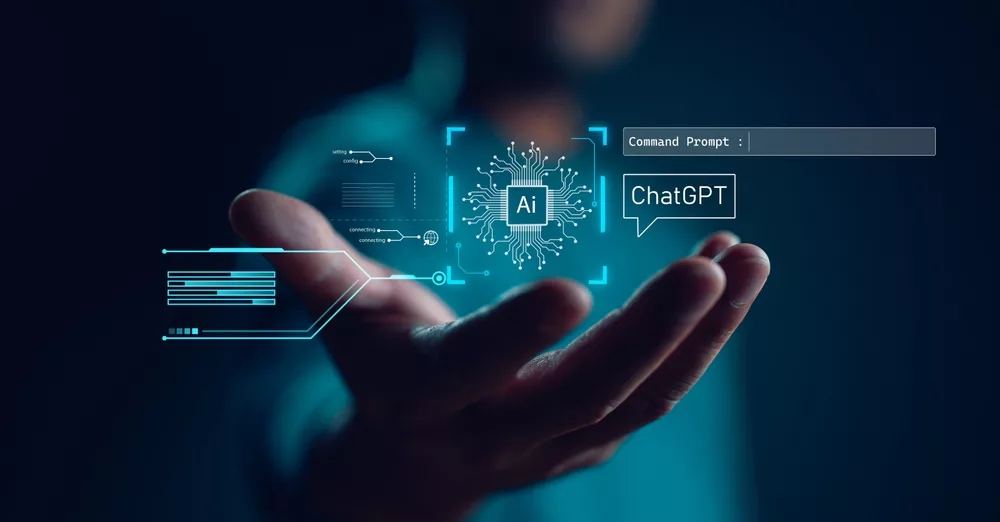
- Artificial intelligence is rapidly transforming our world, yet its centralized nature raises significant concerns regarding transparency, ethics, and accountability.
- However, not all companies building them at scale understand their inner workings and potential risks.
- Decentralized AI—that is, AI models built on or incorporating blockchains—offers a sunlit alternative to proprietary black box Centralized AI models while solving the hard technical problems that accompany this technology boom.
- Read more Crypto Council Explainers.
AI Transparency and Accountability
Centralized AI models are black boxes. These models are opaque by design, making it impossible to understand the inner workings or how outputs are generated. This opacity prevents regulators from effectively overseeing these systems, and prevents the public from knowing if they can trust the models. Decentralized AI, however, uses public blockchains and open-source protocols, ensuring that AI models are transparent and their decision-making processes accessible to review and update. This transparency allows users and regulators to understand AI behavior, promoting accountability and trust, and enabling faster, more iterative innovation based on user feedback and regulatory boundaries.
Reducing Training Bias and False Outputs
Bias and false outputs are prevalent in centralized AI due to inaccurate, incomplete, or selective training data and opaque models. Decentralized AI models, built on the transparent and verifiable data sources of blockchains, can reduce these issues. By grounding AI training in publicly accessible on-chain data and using decentralized networks for validation and compute, Decentralized AI provides more accurate and unbiased results. Models like Graph Neural Networks (GNNs)—trained on complex relational graph data, like blockchain transactions across wallets and smart contracts—can train and continuously update centralized AI models like Large Language Models (LLMs), fostering data transparency and improving model learning. This can reduce biased outputs and the incidence of hallucinations (false or fabricated outputs), improving the quality of AI models overall.
Ethical Data Usage and Model Training
Centralized AI models frequently face criticism for unethical data usage, including training on proprietary data without permission. Decentralized AI leverages public on-chain data, ensuring that data usage is ethical and consent-driven. Training AI models with public, verifiable data sources respects data privacy and avoids legal pitfalls associated with unauthorized data usage. Additionally, Decentralized AI can incorporate user-owned data vaults, wallets, or smart accounts, empowering individuals to control and monetize their data while maintaining data privacy.
6 Practical Applications of Decentralized AI
Decentralized AI addresses transparency, correct outputs, regulatory oversight, and bias. It also solves technical barriers and externalities caused by Centralized AI models.
- Lower Energy Demands: Demand for compute power and electricity has skyrocketed due to AI, requiring more constant, reliable flows of electricity. To meet demand, US energy companies are extending lifespans of coal power plants that were set to be phased out. Georgia is now projecting an energy demand 17 times higher over the next decade. Decentralized AI can distribute the computational and energy load across multiple, geographically distanced nodes, reducing acute local energy demands that lead to shortages.
- Cybersecurity and Network Resilience: Centralized AI black box models are single points of failure, making them attractive targets for cyberattacks as more global cyber infrastructure relies on AI. Blockchain networks supporting Decentralized AI models like GNNs can monitor on-chain activities and detect anomalies in real-time, similar to advanced cybersecurity measures by firms like CrowdStrike. This decentralized approach ensures AI systems are more robust and less vulnerable to attacks. Moreover, the myriad of nodes run on blockchains turn the single point of failure model into multiple sources of resiliency.
- Preventing Walled Garden 2.0: Like the App and Play Stores, Centralized AI models are platforms for new companies and software to build upon. Recognizable by the 30% fee imposed on developers launching on those platforms and in-app payments, centralized models could lead to Walled Garden 2.0. Decentralized AI, built on-chain, lowers these barriers and fosters a competitive environment for builders, breaking down the monopolies of centralized platforms. By enabling startups to deploy AI solutions without these prohibitive costs, we can boost U.S. innovation and GDP.
- Greater Capital Market Efficiency and Transparency: Decentralized AI can enhance investment strategies by providing transparent and data-driven analysis, reducing reliance on opaque proprietary algorithms. Graph Neural Networks (GNNs), which are well suited to track on-chain trading data (and incorporate off-chain signals), provide predictive price and market sentiment analytics. As more Tokenized Real World Assets (RWAs) come on chain, these market efficiencies can extend into the traditional capital markets as well. This transparency builds trust with users, investors, and regulators, ensuring a more stable and secure financial ecosystem.
- On-Chain Marketing: AI-driven marketing models can target users based on their on-chain behavior, such as NFT trades and positions. This personalized approach can increase user engagement and adoption of new protocols, driving growth in the blockchain ecosystem.
- Social-Fi and Gaming: Decentralized AI can analyze user behavior within Social-Fi applications, improving user experiences and engagement. Decentralized social networks can benefit from AI models that understand and predict social interactions, facilitating better community management and content recommendation, creating a better user experience.
Decentralized AI – looking ahead
As Artificial Intelligence booms, we have a chance to make different choices than those that led to the era of centralized power, opaque code, and walled gardens ushered in by tech oligopolies. Decentralized AI promotes transparency, reduces bias and misinformation, ensures ethical data usage, enhances cybersecurity, and can reduce demand for fossil fuels. Policymakers and industry leaders must recognize the necessity of Decentralized AI and support its development for a safe, transparent, ethical, and competitive technological future.
Policymakers must establish regulatory frameworks that encourage the adoption and development of Decentralized AI technologies and the cryptocurrency ecosystems on which they are built. There is a new paradigm in crypto policy. Washington needs to account for crypto’s role in AI. Industry leaders must invest in and promote decentralized AI platforms, fostering a collaborative and transparent AI ecosystem. Together, we can ensure AI innovation remains ethical and transparent, benefiting society overall.